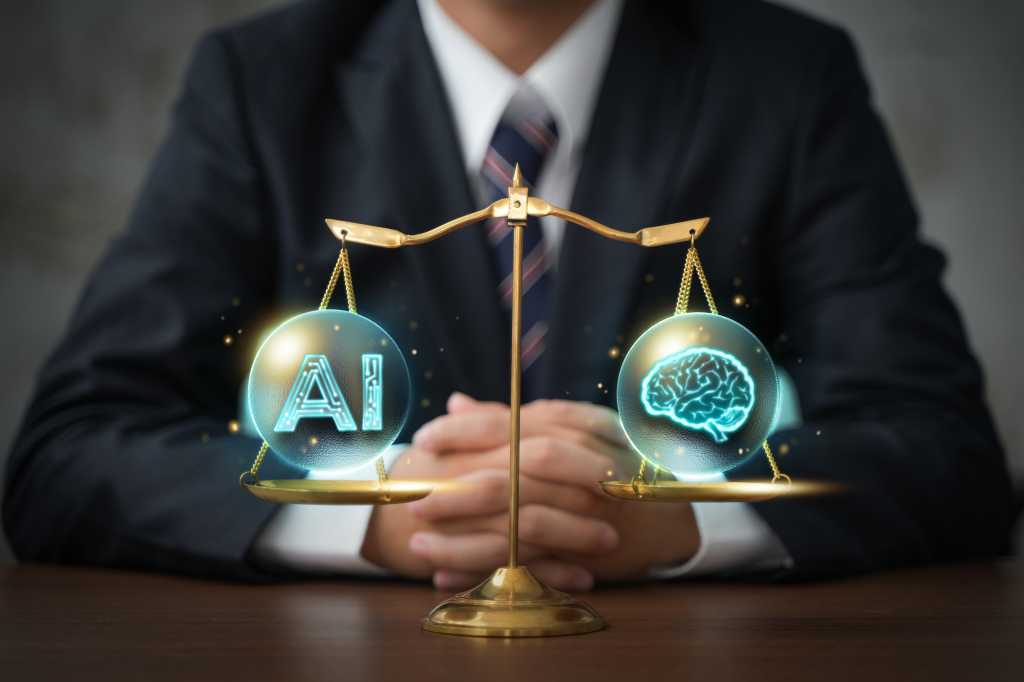
“We are very LLM agnostic, in that we’re able to look at the different tasks and see which one is right for a particular task. For image analysis, or the multimedia analysis, we’re using the latest and greatest, such as the ChatGPT Omni. It’s unique in having capabilities for drafting [client-privilege] log lines. Depending on the data, we’re shifting between GPT-4 or GPT-3.5 Turbo.
“We’re actually looking at where we’re getting reasonable performance and comparing that to things like costs.”
Is price an issue you consider when adopting a model? Mahoney: “Different LLMs have very different price points. For some of our data sets, the way GPT 3.5 Turbo is performing log lines is actually quite good. So, we wouldn’t want to spend the extra money on GPT-4 there.
“On the small language model front, I’d say we’re doing tuning rather than a separate small language model for each application…. We’re taking an existing model — but where we have an industry that might look very different than what that model was built on — [and] we’re doing some fine tuning on top of that to introduce the model to a dataset before it starts making predictions on it.”
So, essentially, some LLMs are better at some tasks than others? Mahoney: “Some language models are better at certain tasks in summarizing or pinpointing whatever it is. Ideally you have a workflow with six steps and you’re using a different LLM at different steps. You never know who’s going to emerge tomorrow and being better at X or Y.
“We’ve been using OpenAI [LLMs] before it was publicly launched. And we’ve been testing Meta and Claude and using the ones that we think make the most sense for a particular task.”
Data scientists and analysts, prompt engineers — what roles do you have or have you added to address your LLM needs? Swansburg: “For the work CJ does, and the work we do, the larger the data set, the more the need for data scientists. So, he does work with data scientists on his side.
“On my side, in terms of prompt engineers, we have good software developers that can do that for you. We have people who are pure developers, and we have people who sit in the middle that we call ‘legal technologists.’ Those are the translators who take client and lawyer requirements and feed those back and do the customization to the platforms we build.
“We don’t have any data scientists yet, because we use discrete data sets. So it’s more about being able to engineer the prompts — and the team we have now has been able to do that on the developer side. As we grow, and right now we’re recruiting another half-dozen developers, we will get more nuanced and look for people with prompt engineering experience and building APIs with LLMs and other tools.
“So, it’s constantly changing.”
Are you’re mostly using proprietary rather than open-source models? Mahoney: “Right now, we’re just using proprietary models and plugging them in and testing them — OpenAI being the more common example. We’re building things through prompts like contract determination dates to extract that data we need and building bundles of questions that will be generated based on the automatic determination of what the system in ingesting. All of that is being tested now.
“Some of them are really expensive. Something like ChatGPT is very accessible. Even the enterprise models can do the trick, and they’re accessible and affordable. “
If legal departments and law firms were already using AI and ML, why is ClearyX needed? Swansburg: “We’re trying to build a model that’s a lot less expensive than contract management software…and to have much higher quality than a lot of providers and provide a service.
“A lot of companies don’t have people to own and operate these programs. So, they have shelfware. They buy a contract lifecycle management tool, and it take three years to get their return on investment; then people don’t use it because it’s not custom designed. So, we’re trying to build custom solutions for clients that work the way they work, and that are affordable.
“We’re not venture capital owned. We’re owned by the partnership, so we’re able to build things in the right way. We’re not just serving clients of the Cleary law firm; we also have a mandate to get outside clients.
“We started thinking we weren’t going to be a development shop. We were going to use existing solutions and weave them together using APIs, but a couple things happened. The tools on the market weren’t doing what we wanted them to do. We weren’t able to customize them in the nuanced way that made clients actually delighted to use them.
“The other is the ubiquity of AI, and the ability to customize them is way easier than it was three years ago. So, over the last eight months or so, we’ve been able to pivot to something that allows us to customize it more easily and collaborate with clients to figure out how they want it to work.”